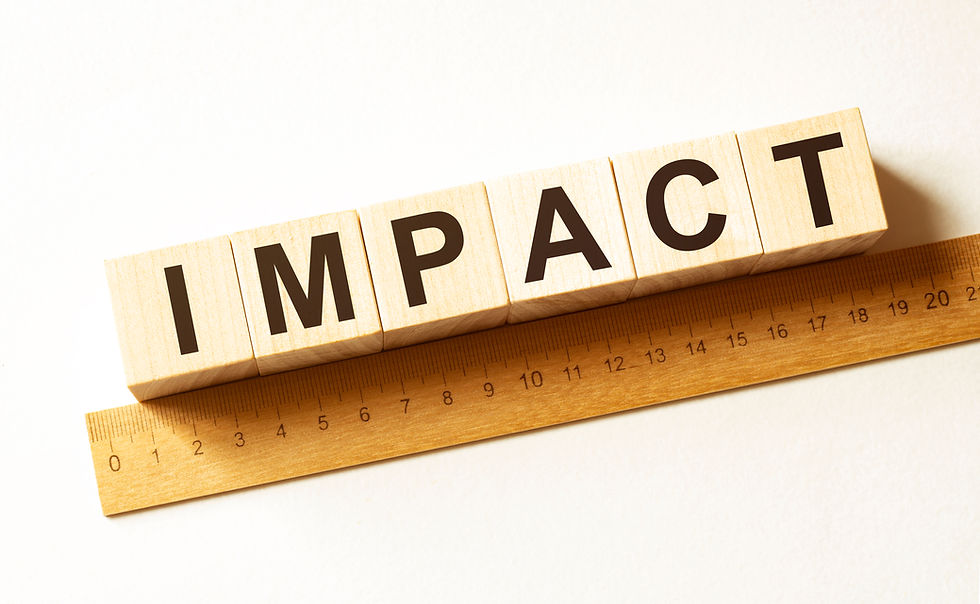
In this blog post, we explore the significance of Rigorous Impact Evaluations (RIEs), what they are, how they can help bridge the gap between academia and applied evaluations of international development initiatives, and the next steps needed towards building a systematic body of evidence in international development. The blog post is structured as follows:
Section 1 defines Rigorous Impact Evaluations
Section 2 summarises why we need Rigorous Impact Evaluations
Section 2 highlights the ongoing gap between academia and the development sector
Section 3 examines the next steps for RIEs
1. What are Rigorous Impact Evaluations?
A rigorous impact evaluation (RIE) is a particular type of evaluation that seeks to measure what would have happened to project participants in the absence of a development project (intervention).
RIE is the umbrella term for various types of evaluations measuring causality: a) experimental, and b) quasi-experimental methods .
RIE is the umbrella term for various types of evaluations: a) experimental- b) quasi-experimental (and non-experimental) methods that can establish causality. All use a counterfactual approach (a so-called control or comparison group) to uncover the impact of a development project. This differentiates RIEs from traditional evaluation methods, such as pre-post evaluations, where data is collected only on project participants, before and after the project. These traditional evaluation methods suffer from two main biases, selection bias, and confounding, which RIEs aim to reduce.
Selection bias is a research error that occurs when the chosen sample isn't representative of the larger population. It happens when samples are not randomly selected, individuals self-select into studies, or when only “project survivors, i.e. those that did not drop out” or convenient groups are considered. This bias can distort study results, leading to under or overestimation of the real impact.
Confounding is when a third, unaccounted for variable distorts the
apparent relationship between two other variables. This bias can again distort study results, leading to under or overestimation of the real impact.
There is a perceived hierarchy in RIEs. Compared to full experiments, quasi-experiments and and more so non-experiments may have a lower internal validity (but a higher one compared to non-counterfactual evaluation methods) because they lack the rigour of random assignment. This makes it more challenging to entirely rule out the influence of confounding variables and selection bias. We further describe the differences in approaches below. Also, have a look at this video that explains RIE in a simple and visual manner.
2.1. RIE Type A: Experiments
The “gold standard” of RIEs is an experimental evaluation, a so-called Randomised Control Trial (RCT), which originated in the field of medicine. In experimental evaluations, participants are randomly assigned to either the treatment group (those who receive the intervention) or the control group (those who do not receive the intervention) before the start of the intervention. Random assignment helps ensure that the groups are comparable at the outset, largely reducing the influence of selection bias and confounding. An RCT must already be planned before the start of the development project to ensure randomisation. A well-designed RCT can thus reduce the issues of selection bias and confounding to determine the real impacts of the development intervention on its participants.
Figure 1: Randomised Control Trial
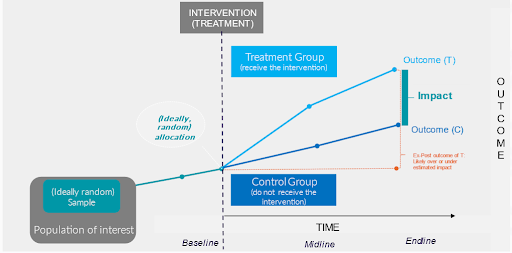
2.2. RIE Type B: Quasi-Experiments
The main difference between experimental evaluation and quasi-experiment lies in the use of random assignment. In quasi-experiments, participants are not (entirely) randomly assigned to the treatment or comparison groups. This may be because random allocation (e.g. of project participants) was not possible or treatment and control groups are found to be statistically different at baseline. Quasi-experimental methods may include
Difference in Difference (DID) approach,
Regression Discontinuity designs (RDD)
A common quasi-experimental design is a Difference in Difference (DID) approach, which estimates the average changes in outcome between the treatment and comparison group over time, thus requiring baseline and endline data for both groups. The baseline data is collected to understand the potential differences and thus changes over time between the two groups. This can be combined with statistical matching of the two groups along the lines of observable characteristics at baseline to ensure that treatment and comparison groups are as similar as possible.
Figure 2: Difference-in-Difference Analysis
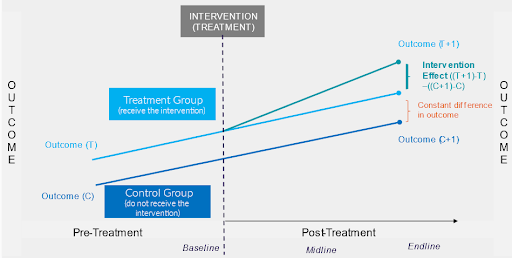
When experimental and the above-described quasi-experimental approaches are not feasible, researchers can also apply non-experimental methods to draw causal inference such as:
Matching Methods such as Propensity Score Matching Analysis (PSA),
Inverse Probability Weighted Regression Adjustment (IPWRA)
Instrumental Variable (IV),
Synthetic Control
Interrupted Time Series, and so on.
Some of these methods can be applied when only post-project data on the treatment and comparison groups are not available (ex-post), because the evaluation was not planned before the start of the project.
2. The Importance of Rigorous Impact Evaluation
“Most interventions don’t work, most interventions aren’t evaluated, and most evaluations are not used.” (White, 2019)
RIEs play a crucial role in determining what works, what does not work, and why, in international development by answering key questions:
Assessing Impacts: RIEs allow organizations to make informed, evidence-based decisions to determine which development projects achieve their intended impact, which do not, and why, ensuring resources are directed where they can make the most difference
Learning from Experiences [Understanding Failures]: RIEs help organizations learn from both their successes and failures, guiding better decision-making in subsequent projects, and avoiding the repetition of past mistakes
Supporting Policymaking: RIEs offer evidence for policymakers, informing decisions about which initiatives to endorse and expand, and promoting evidence-based policy development.
Enhancing Accountability and Transparency: RIEs establish transparency and accountability in project evaluation, ensuring responsible resource allocation.
Fostering Innovation: RIE findings inspire innovation by uncovering unexpected insights.
Thus, by embracing RIEs, organisations can make more informed decisions, achieve greater accountability, and ultimately have a more meaningful impact on the communities they serve.
3. The Gap
There is a gap between impact evaluations applied in academia and those used for real-world development projects.
Rigorous Impact Evaluations are a part of the evolving landscape of evidence-based approaches in international development, comprised of four waves: (1) outcome monitoring, (2) impact evaluation, (3) systematic reviews and (4) knowledge brokering (White, 2019). RCTs (RIE type A) have increasingly gained traction in international development, exemplified by the establishment of organizations like J-PAL, IPA, and the World Bank's Development Impact Evaluation Initiative (DIME). Notably, the 2019 Nobel Prize was awarded to Duflo, Kremer, and Banerjee for their experimental approach to poverty alleviation. This uptake of RIEs, especially of RIE type A, can be observed below..
Figure 3: The Uptake of RIEs in international development
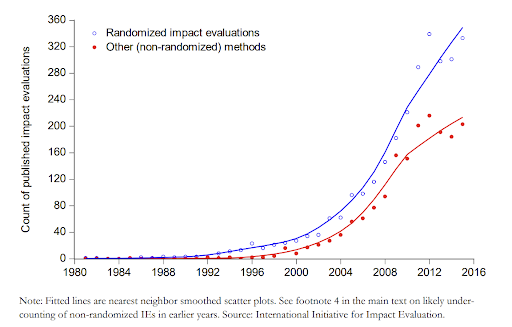
Source: Ravallion, 2020.
Yet, most of this uptake in RIEs, especially but not only randomised impact evaluations (type A), is still largely dominated by academia. Between 1990 and 2015, 65% of RIEs were published in health journals, whilst RIE publications in social science journals have also risen considerably in the last two decades (Sabet and Brown, 2018). Whilst RIEs commissioned by NGOs, Governments and international development organisations to evaluate projects and programmes (so-called real-world impact evaluations) have slowly been increasing since the early 2000s (Cameron, D.B., Mishra, A. and Brown, A.N., 2016), they are still far less common.
This is at least in part because randomised impact evaluations must be planned at the programme's conception to ensure that treatment and control groups are allocated randomly. Yet, in international development, evaluations are rarely planned during the project/programme planning phase. Instead many organisations commission an external evaluation at the end of the implementation phase. At that stage, it is too late to introduce an experimental design. Another factor to consider is that in real-world settings, it may be impractical or unethical to randomly assign participants to different groups, making pure experimental evaluations unfeasible.
There are many more reasons why RIEs may fail in practice. For one, project implementers may prefer to remain flexible during project implementation and adjust the treatment or the target groups. Other reasons include resource constraints that development organisations face, as well as a lack of time, lack of methodological knowledge and funds, insufficient and untimely planning, and high costs associated with RIEs. Lastly, many RIEs fail because of a small number of project participants, resulting in difficulty in allocating a meaningful control group (see for instance Krämer et al. 2021, GIZ/GOPA 2023). To better understand when RIEs can be applied, have a look at this blog post.
2.3.1. Bridging the Gap? Mixed-Methods Quasi-Experiments
To bridge this gap between impact evaluations used in academia and real-world development projects, some have proposed mixed-methods quasi-experiments (RIE type B), which are less rigorous than an RCTs (RIE type A) but still offer more accuracy and validity in measuring impact than traditional ex-post evaluations (see for instance Woolcock, M., 2019; Guijt and Roche, 2014; Chatterji, M., 2004). Furthermore, some have put into question whether RCTs should continue to be the gold standard (Ravallion, M., 2020). A preference for quasi-experiments is reinforced by an identified need for a more ethical, people-centric approach in development contexts (GIZ/GOPA,2023), revealing a tension between academic prestige, and practical, ethical considerations in project evaluations.
To enhance insights from quasi-experiments, which might lack the rigour of traditional RCTs, the integration of qualitative data through a mixed-methods approach is vital, enriching understanding and contextualizing outcomes.
Mixed method approaches often make use of key-informant interviews, focus group discussions or in-depth interviews with participants to inform the evaluation design and questionnaire before a survey takes place, or to explain and triangulate the findings after a survey is conducted. These could also include other qualitative methods like participatory social network mapping, participatory rural appraisal tools, or visual digital tools.
Recent evaluation literature highlights a shift towards understanding not only whether interventions work but also how, why, and under what conditions they do (White, 2019). This requires delving into the causal mechanisms behind the driving effects. Rigorous Impact Evaluations are much more valuable when both grounded in theory and combined with qualitative methods, to provide a comprehensive understanding of interventions.
4. Next Steps for Rigorous Impact Evaluations
4.1. Developing a Sound Body of Evidence
What happens after an RIE has been conducted is equally important. The findings from impact evaluations have shown that there is in general an 80% rule, meaning 80% of interventions do not work (White, 2019). This makes conducting RIEs to find out and upscale what works in international development even more important. Evidence-based decision-making in international development should be based on a systematic assessment of the existing body of evidence, not a single impact evaluation. RIEs are often criticised for lack of external validity; yet it is important to know that impact will vary by context, population, intervention design and even time (White, 2009). This is where systematic reviews and evidence-gap maps come in. Developing a sound body of rigorous evidence is a bit “like building a mosaic. Each individual piece does not make the picture, but bit by bit a picture becomes clearer and clearer” (Gugerty and Karlan, 2018) on what works and what does not work in development projects, and why.
4.2. Systematic Implementation and Evidence Synthesis
Some development agencies require a statement of evidence from rigorous impact evaluations to support new proposals, and/or how the proposed development project will include the collection of the needed evidence if it does not exist yet. Yet, for the majority of development organisations and governments, RIEs are still commissioned on an ad-hoc, unsystematic basis, and the evidence is not used systematically for informing new development interventions (see our blog post on RIEs in the German development sector). Incorporating systematic review findings into policy and practice therefore constitutes the latest (fourth) wave of the evidence revolution: knowledge brokering or knowledge translation White (2019). The next steps therefore lie in increasing the systematic implementation and uptake of generated evidence from RIEs to make interventions work.
4.3. The role of AI
AI, big data and machine learning can play a role in making RIEs and evidence synthesis more accessible. They may therefore constitute a fifth wave of the evidence revolution (White, 2022; White, 2019). For instance, using satellite data to assess the impact of agriculture interventions may make RIEs more cost-effective. Machine learning can also help in producing systematic reviews and evidence-gap maps, making it easier for policy-makers to use the generated evidence for decision-making.
Others have pointed out that impact evaluations are too often donor-driven, and not embedded in partner governments (Manning et al. 2020). Therefore, rigorous impact evaluations should be embedded in a “broader spectrum of tools available to support policymakers, ranging from evidence maps, rapid evaluations, and rapid synthesis work, to formative/process evaluations and systematic reviews” (Manning et al. 2020).
Whilst there is still much left to do, what is clear is that Rigorous Impact Evaluations have and will play an important role in supporting evidence-based learning in international development.
5. Bibliography
Cameron, D.B., Mishra, A. and Brown, A.N., 2016. The growth of impact evaluation for international development: how much have we learned?. Journal of Development Effectiveness, 8(1), pp.1-21.
Chatterji, M., 2004. Evidence on “what works”: An argument for extended-term mixed-method (ETMM) evaluation designs. Educational Researcher, 33(9), pp.3-13.
GIZ/GOPA 2023. Measuring Results of Skills Development Interventions: Experiences of Impact Evaluations by German, Swiss and Austrian Development Cooperation. Esser, Ursula, Holzaepfel, Franziska, Zamalloa-Huegel, Patricia. Bonn: GIZ. Available at:
Gugerty and Karlan. (2018). Ten Reasons Not to Measure Impact—and What to Do Instead. Stanford Social Innovation Review. https://ssir.org/articles/entry/ten_reasons_not_to_measure_impact_and_what_to_do_instead#:~:text=Resource%20limitations%20can%20doom%20the,unless%20the%20impact%20is%20massive.
Guijt, I. and Roche, C., 2014. Does impact evaluation in development matter? Well, it depends what it’s for!. The European journal of development research, 26, pp.46-54.
Krämer et al. (2021), Rigorous Impact Evaluation: Evidence generation and take-up in German development cooperation, German Institute for Development Evaluation (DEval), Bonn.
Manning, R., Goldman, I. and G. Hernández Licona. (2020) “The Impact of Impact Evaluation: Are Impact Evaluation and Impact Evaluation Synthesis Contributing to Evidence Generation and Use in Low- and Middle-Income Countries?”, WIDER Working Paper, Helsinki: UNU-WIDER. https://doi.org/10.35188/UNU-WIDER/2020/777-4.
Ravallion, M., 2020. Should the randomistas (continue to) rule? (No. w27554). National Bureau of Economic Research.
Sabet, S.M. and Brown, A.N., 2018. Is impact evaluation still on the rise? The new trends in 2010–2015. Journal of Development Effectiveness, 10(3), pp.291-304.
Schmitt, J. (2020). The causal mechanism claim in evaluation: Does the prophecy fulfill? In J. Schmitt (Ed.), Causal Mechanisms in Program Evaluation. New Directions for Evaluation, 167, 11–26.
White (2009). Theory-Based Impact Evaluation: Principles and Practice. 3ie Working Paper 3. https://www.3ieimpact.org/evidence-hub/publications/working-papers/theory-based-impact-evaluation-principles-and-practice.
White, H. (2019) The twenty-first century experimenting society: the four waves of the evidence revolution. Palgrave Commun 5, 47. https://doi.org/10.1057/s41599-019-0253-6.
White, H. (2022) The unfinished evidence revolution: riding the five waves. CEDIL Methods
Working Paper 8. London/Oxford: Centre of Excellence for Development Impact and Learning (CEDIL). https://doi.org/10.51744/CMWP8.
Woolcock, M., 2019. Reasons for using mixed methods in the evaluation of complex projects. Contemporary philosophy and social science: An interdisciplinary dialogue, 147, pp.123-210.
Comments